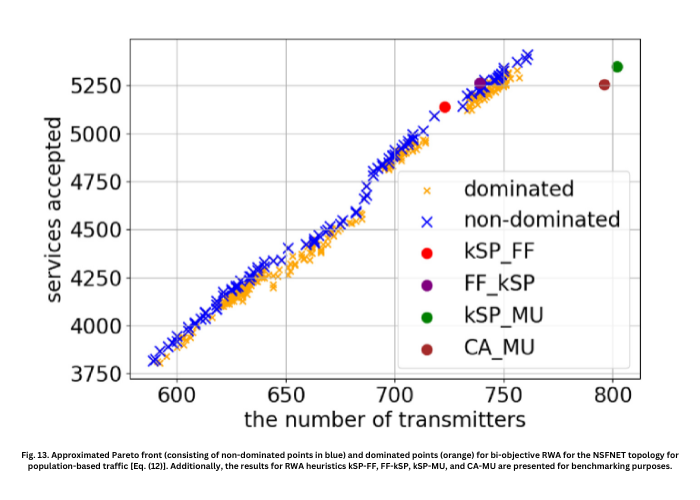
TRANSNET researchers propose novel Multi-Objective Reinforcement Learning for enhanced network performance
Delving in the complexities of optical network optimisation, TRANSNET researchers introduce a cutting edge-reinforcement model, offering a powerful tool for parameter optimisation within optical networks.
Optical networks form a vital backbone of our modern digital infrastructure, enabling high-speed, reliable, and secure communication in our increasingly interconnected world. Given that there is an ever-increasing demand for data-intensive applications, researchers across the world have been carrying out significant work to improve the performance of optical networks. Reinforcement learning (RL), a branch of artificial intelligence, is crucial to this work due to their ability to optimise network performance in complex and dynamic environments.
In this study, the authors tackle the complex issue of optical network performance optimisation, which often requires balancing objectives like maximising throughput, minimising latency, reducing costs, and enhancing resilience. Unlike traditional methods that focus on a single objective or performance goal, this groundbreaking approach places an emphasis on the simultaneous optimisation of multiple objectives – a critical factor in the design and operation of modern optical networks.
Drawing upon real-world case studies, the authors tackle the challenging “routing and wavelength assignment (RWA)”, proven to be an NP-hard problem in optical network literature. Thus, they propose a novel approach for solving the multi-objective RWA problem in optical networks under dynamic traffic conditions. In summary, the model provides optimal results concerning the allocation of routes and wavelengths for each demand, the number of accepted services, the number of transmitters, and network availability. The result is an approximated Pareto front offering a spectrum of solutions, enabling network operators to make decisions that align with their specific objectives.
In comparison to existing methods, the authors proved this approach to be equally effective in finding optimal solutions, but with the added benefit of providing multiple optimal values. An additional advantage is the reduction in computational cost in machine learning model training, making this approach more energy efficient.
The overall insights from this study could potentially revolutionise network design, operation, and growth strategies, making reinforcement learning a game-changer for dynamic network optimisation.
About the paper
The work, to be published in the August 2023 issue of Journal of Optical Communications and Networking, is led by TRANSNET investigator Professor Seb Savory with leading author Sam Nallaperuma, both from the University of Cambridge. Full paper can be accessed here.
"Interpreting multi-objective reinforcement learning for routing and wavelength assignment in optical networks," J. Opt. Commun. Netw. 15, 497-506 (2023)
Sam Nallaperuma, Zelin Gan, Josh Nevin, Mykyta Shevchenko, and Seb J. Savory